Expert Tips for Winning Daily Fantasy Tennis
Learn the essential strategies for winning at Daily Fantasy Tennis during the 2024 French Open. Ideal for both seasoned players and newcomers, this video provides comprehensive insights to elevate your tennis DFS game this summer. Discover how to build high-upside lineups, optimize for winning outcomes with simulations, and navigate the complexities of player projections. Learn how to outperform competitors by balancing raw scoring potential with ownership considerations, and leverage detailed player research for maximum ROI.
The 2024 Tennis French Open is here and there is no better time to dive into tennis DFS. This tournament kicks off the best part of the tennis season, and it's an awesome DFS sport to get into this summer. Tennis DFS has awesome sweats, and the Grand Slams have killer prize pools, and in this video, I'm gonna give you everything you need to know to beat it.
My name is Jordan Chand, I'm the head coach here at SaberSim, and this video is a breakdown of everything you need to know to build winning tennis DFS lineups. I'll walk through the two key components that make a winning tennis lineup. teach you an effective process you can use on sabersim, and show you how you can add value with your research.
Just like any DFS sport, to take down tennis tournaments, you need lineups with upside, and in tennis DFS, there are two parts to this. First, you need a lineup that is capable of putting up a high score. And this sounds simple, but this is actually a hard problem to solve. Average projections and lineups built to maximize average projection are misleading.
A player's average projection is a summary of the full range of outcomes of that player, but equating upside to average projection is a mistake. Averages, for example, assume that every favorite on the slate has higher upside than every underdog, but that's clearly not the case. Many underdogs have the same upside as favorites when they can pull out a win.
Optimizing based on average projection also assumes that the average outcome happens 100 percent of the time, and in tennis, the average outcome for a player is often actually a very rare event. The average splits the difference between a player's winning outcomes and losing outcomes, and ends up in no man's land.
A projected score that happens very rarely. If you've used a traditional optimizer to build tennis DFS lineups before, you probably already know this. An underdog with a 20 percent chance to win will literally never show up in your lineups, even if they're likely to be in the winning lineup if they win, and players with good projections relative to their salaries will get jammed into 100 percent of your lineups, even if that isn't in line with their probability of winning, or their likelihood of showing up in the winning lineup in your contests.
The tools most people use to solve this problem with optimizers and average projections only make the problem worse. Randomness assumes a normal distribution for players centered around their average projections. In other words, a bell curve where the most common outcomes are all around the average.
And while randomness will start to get other players showing up in your lineups, the distribution used here is not in line with reality. Tennis players have bimodal distributions, two roughly normal distributions based around the outcomes where they win and the ones where they lose. And setting min and max exposures to force players into your lineups is just restricting the optimizer on one player and then optimizing for the average projection for the rest.
If you set a minimum 20 percent exposure on that underdog with a 20 percent chance to win. You're just going to get the five best players in the rest of the lineup. What you really want is an even distribution of the other players on the slate at the rate at which they themselves are likely to win.
And optimizing for a ceiling projection instead of the average projection has the opposite problem. Because in that case, you're just assuming everyone wins, which doesn't make sense either. This approach is going to way overvalue underdogs. Again, painting a picture of the slate in your lineups that isn't realistic.
To be successful in Tennis DFS, we also need to understand how players win when they win. To take down large field tournaments, it's not always enough to just have six players that win their match. Players unlock their full upside by dominating their match. Winning in straight sets, racking up aces, breaking their opponent's serve, and so on.
An expensive favorite will have ways of winning a close match that doesn't actually end up giving you enough upside to make it worth it in your lineups. A simulation of tennis matches solves these problems directly. By playing out each match on the slate thousands of times, we can build out the full range of outcomes of each player.
Understanding both how often they win and lose, but also what they're scoring when they win. Then, we can combine simulations of each match together to put together scripts of how the entire slate plays out, and build the best possible DFS lineup for each of those different outcomes. With this approach, each of our lineups has the upside needed to win, because it's the best possible lineup to win.
for a given simulation of the slate, and players appear in our lineups at the rate at which they are optimal for the slate. This is exactly how SaberSim works. We use the vegas lines to set the probabilities of each player winning their match, plus use historical data to account for playstyles, how aggressive players are, and what types of wins they're likely to have.
When you press build, each lineup you get back on SaberSim is the best. best possible lineup for a given simulation of the entire slate, and we build thousands of them in just a few minutes. To get this process going here, let's go ahead and build some lineups here. For this example, using the French Open from last year, we don't have simulations up just yet at the time I'm recording here, but to get this going here, we'll assume we're building for a relatively large field 150 max tournament.
I'm not going to make any adjustments ahead of here. And I'm just gonna go ahead and press build. Again, what this is doing is essentially simulating out the opening first round from last year 5, 000 times, building the best possible lineup for 5, 000 different ways that the slate might play out. But raw scoring upside is not the only thing that makes a profitable Tennis DFS lineup.
You need lineups that can outscore your opponents. We're not trying to score an arbitrary number of points with our lineup. We are trying to outscore the lineups our opponents play. This means fantasy points scored by our players have more value the fewer lineups they show up in in our contests. A lineup that has a huge score isn't very useful to us, if a ton of the lineups we're playing against also all have the same players.
But there is a balance here. Favorites and players with big upside potential are going to be popular in DFS contests, so we often have to make trade offs by sacrificing scoring upside to get lower ownership. Striking this balance correctly is difficult. We don't want to give up too much on either side.
We can solve this problem with a DFS contest simulation. A contest sim uses a projected field of lineups that are expected to be in your contest and competes a given lineup that you're considering playing against them using simulations of how the matches on the slate can play out. Each lineup gets scored for how it would perform in a given simulation.
Winnings are assigned to each lineup based on the payout structure of the contest, and this process is repeated a hundred thousand times, allowing you to calculate an average ROI of that lineup. You can then repeat this process for each lineup you're considering. In this case, the optimal lineups that you've built from our simulations to identify the most profitable ones to play in your contests.
These lineups strike the correct balance between raw scoring upside and ownership by testing out how they would perform in the actual contest that you're playing. Now that the contest sim is complete, we can sort the lineups by the ROI in that simulation, identifying the lineups most likely to be profitable when you play them into your contest.
At this point in the process, we've identified the most profitable lineups from our simulations to enter. But, no model is perfect, and this is the perfect opportunity to review and revise your lineups to make any adjustments needed. But before we do that, let's set up some guardrails. The first thing I'm gonna do is filter out unprofitable lineups from the contest simulation.
Just because a lineup showed up in our pool here doesn't necessarily mean it'll make us money if we play it into the contest. So let's filter out all the lineups that had a negative expectation here. We'll add a filter and say, only show lineups where the ROI was greater than zero. Next up, we can add a couple aggregate metrics to get a sense of what the characteristics of the lineups in our pool actually are.
Here we'll add a couple aggregate metrics. What I like to do here is set a metric that says what is the minimum ROI. So this will show us what's the lowest ROI lineup in our set of one 50 here, and also the average ROI. Together, as we're making changes to the lineups that show up in our top 150, this will give us a good sense of how deep into our pool we're going.
So the lowest ROI lineup we have in our 150 lineup set right now is 38. 9, and on average, if you average those ROIs across all 150 here, it's 54. 4. The backstops we've set up here will make sure we're only playing lineups that showed up as profitable in the contest sim, and let us keep an eye on how deep we're going into our lineup pool.
When it comes to making adjustments and reviewing and revising at this step, there are a few columns in SaberSim that are particularly useful. The first is the leverage column, which is your current exposure minus the ownership projection. Sorting this column in both ascending and descending order will help you identify the players you're taking the biggest stands on relative to the field.
These are the players that are poised to make you the most amount of profit when you're right, but they're also the players that you are exposed to the greatest amount of risk on when you're targeting your research. The players that have the highest and lowest leverages are the players that you should be focusing on.
The ownership projection lets you know what percentage of lineups in your contest we expect a given player to appear in. Getting closer to the ownership Projection with your exposure will reduce the risk you're taking on a given player. And being closer to ownership overall is a more neutral stance.
However, the players that you are different from on your ownership projections are the players that you stand to gain the most from when you're right. So Francis TFO here is our highest exposed player relative to ownership. If he won this match, and particularly if he dominated this match, that would be very beneficial to us.
But if we didn't want to take as much risk here with our portfolio, we could get closer to his exposure. by reducing our exposure of 62 closer to 18. 2 percent. The pool exposure is the percentage of lineups in your pool that have a particular player in them. And this number can be useful when we remember that each lineup in our pool is the optimal for a given way that the slate can play out.
The pool exposure is therefore an approximation of how likely a player is to be in the winning lineup. If you want to take a stand on a player or reduce your stand on a player, you can use this number to contextualize how much exposure you should get. If you like an underdog that has very low ownership for example, you can check to see what that player's pool exposure is and match it with your exposure.
Going back to the Francis TFO example here, you If we wanted to reduce some of our risk here, we could get a little bit closer to the pool exposure, which is a more neutral number of how likely that player is to be in the optimal lineup. For example here, set my maximum exposure to 36%, which gets us to around double the field on this particular player and matches our exposure in our 150 to the pool exposure we have in our overall set.
You can make as many changes as you want at this point, but once you're satisfied with your lineups, you're ready to enter them into your contests. All it takes here is to click Save to My Contest here, downloading your entries file from the site, uploading the entries file, and then clicking Save to My Contest to get your lineups entered.
Tennis DFS is one of the most fun DFS sweats out there, and the French Open is a great spot on the calendar to dive in. By using simulations of each match to build sets of lineups with upside, contest simulations to identify the most profitable ones to play, and a process of reviewing and revising your lineups based on your research, you'll be way ahead of the competition, trying to limp their way along with average projections and traditional optimizers.
But don't just take it from me. This time last year, I interviewed SaberSim data scientist Will. and DFS Pro and Tennis Expert Sheets on their insights about how to beat tennis DFS. What I've shared with you today is a summary of the big takeaways from that interview, but if you're ready to go one step further with your tennis DFS expertise, you can check out the full video here.
And make sure to subscribe to get notified every time I post a new video. I'll see you in the next one, and good luck in the French Open.
related playlists
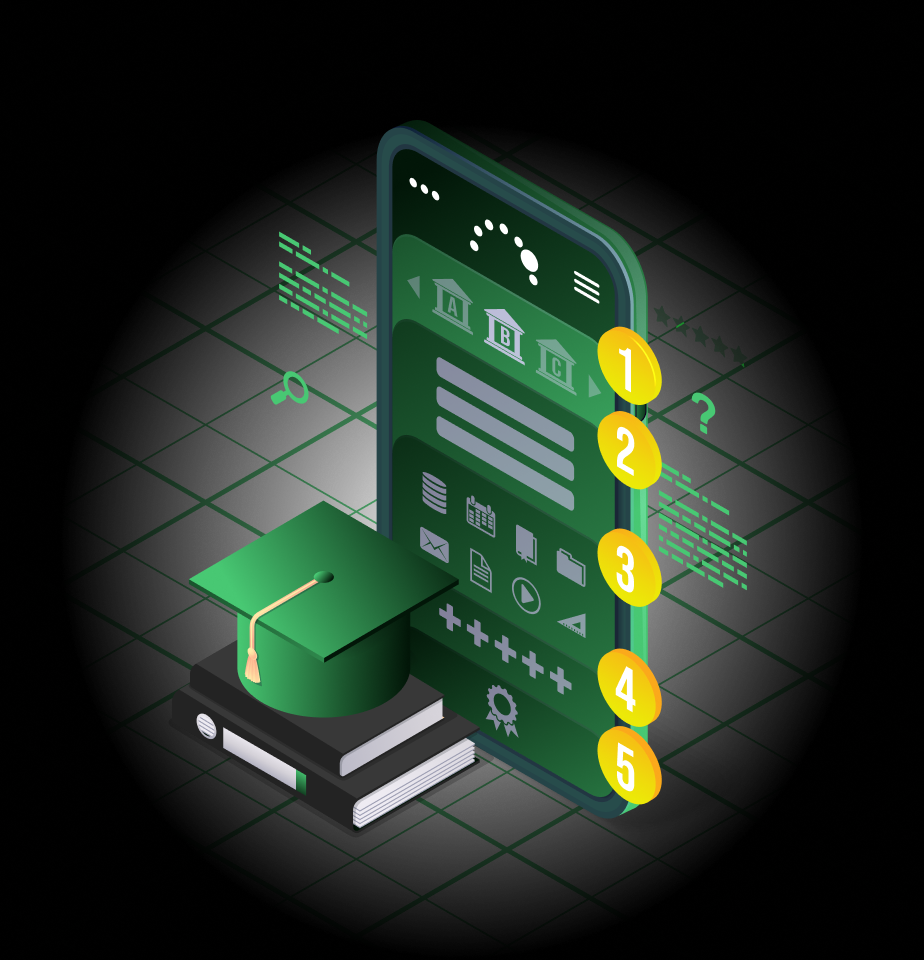
Unlock Expert Knowledge with SaberSchool
Master strategies, insights, and tools to outsmart the competition. Sign up for SaberSim Premium today!